Go to CalR, a web application for indirect calorimetry analysis.
The CalR project:
There is a critical lack of standardization for indirect calorimetry experiments to measure body composition. Currently, the same dataset can produce alternative conclusions based on the analysis method chosen by the investigator. This topic has been discussed by multiple review articles (see Arch, J., Hislop, D., Wang, S., and Speakman, J.; International Journal of Obesity 2006, or Butler A.A. and Kozak L.P. Diabetes 2010). These and other commentaries have squarely laid the blame on inappropriate statistical analysis as contributor to the reproducibility crisis in nutritional and obesity research. We have attempted to fix this specific problem by developing tools that are relatively quick and easy to use. Using our understanding of mammalian metabolism, the limitations and advantages of indirect calorimetry methods, and common analysis pitfalls, we developed a free online statistical software package for the analysis of indirect calorimetry experiments–CalR. This program is made easily accessible to the public as a web tool (CalRapp.org). The software uses a graphical user interface which obviates the need for users to have programming experience. Our tool has been used to analyze more than 24,000 experiments worldwide (Mina et al Cell Metabolism 2018). It has standardized the analysis methods and file formats allowing for greater portability, transparency, and reproducibility in obesity research. Our tool has quickly become the worldwide standard analysis method. These methods are helpful for the community of metabolic researchers working in rodent models, however once completed, these tools may also provide a useful framework to help analyze human metabolic research.
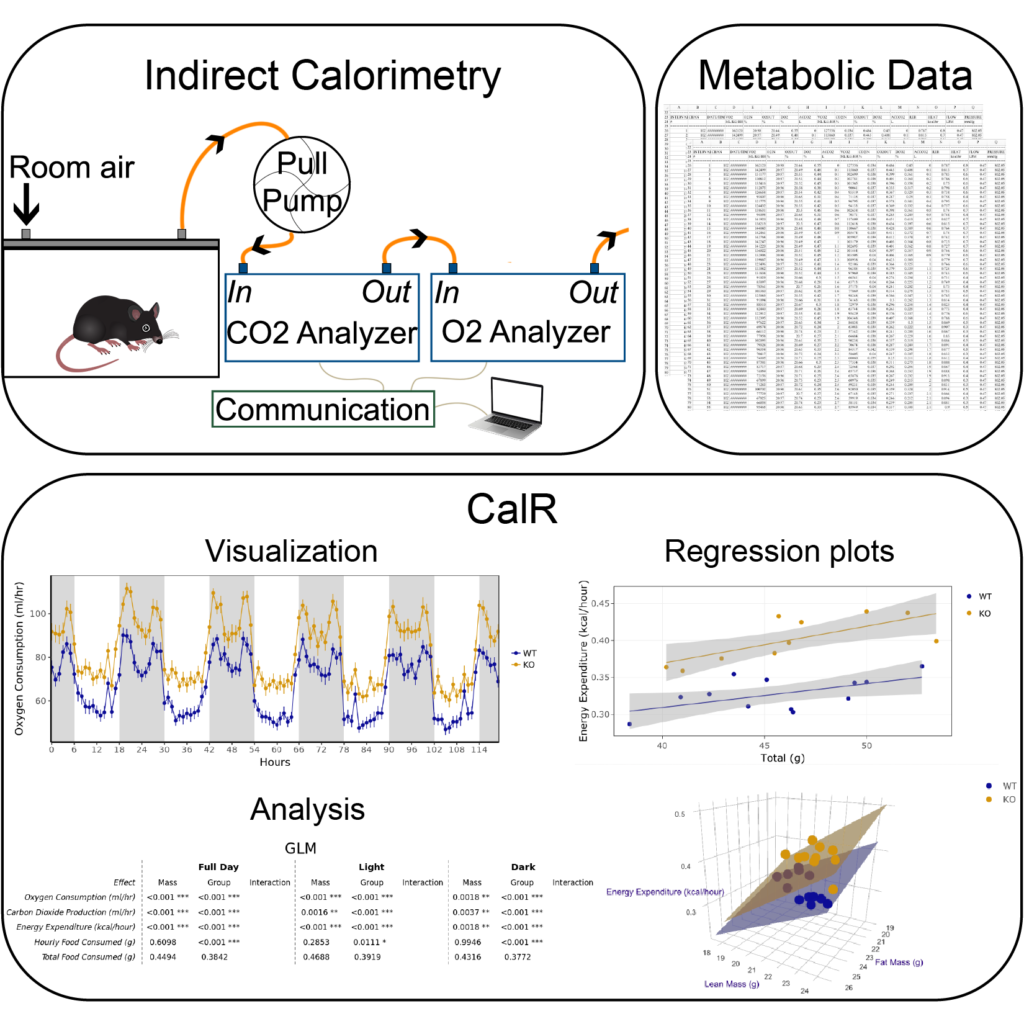